Institute for Nonlinear Science University of California San Diego La Jolla CA 92093-0402 Dated. This procedure simultaneously quantifies categorical variables while reducing the dimensionality of the data.
Pdf Principal Components Analysis With Nonlinear Optimal Scaling Transformations For Ordinal And Nominal Data Semantic Scholar
The goal of this paper is to dispel the magic behind this black box.
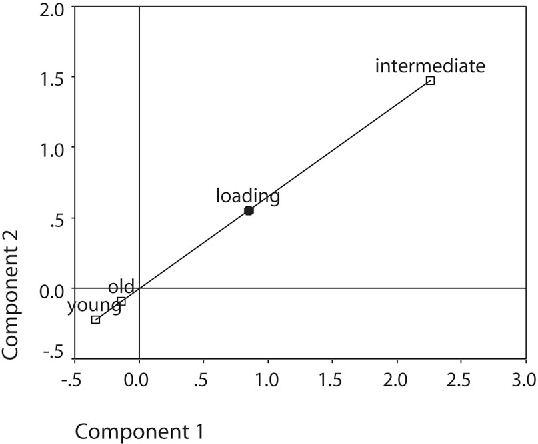
. One of the most commonly used methods to reduce the dimension of data and reveal hidden patterns is the principal component analysis PCA. Since the early 1930s classical statistical methods have been adapted in various ways to suit the particular characteristics of social and behavioral science research. Suppose that some CATPCA-analyzed ordinal or dichotomous variable A is higher value for those who agree in that question asked.
NLPCA is a more flexible alternative to linear PCA that can handle the ana. Without making any assumptions about the measurement levels of the variables. The authors provide a didactic treatment of nonlinear categorical principal components analysis PCA.
Nonlinear principal components analysis. Journal of Personality Assessment 941 1225. Then if A is positively loaded by the component then those who have a higher component.
This article is set up as a tutorial for nonlinear principal components analysis NLPCA systematically guiding the reader through the process of analyzing actual data on personality assessment by the Rorschach Inkblot Test. This article is set up as a tutorial for nonlinear principal components analysis NLPCA systematically guiding the reader through the process of analyzing actual data on personality assessment by the Rorschach Inkblot Test. Similarly the tutorial describes the use of bootstrap confidence interval to test the significance of the factor loadings but the Bootstrap Confidence Ellipses option under the Save menu seems disabled or I cant seem to activate those.
The subscales of job. Nonlinear principal components analysis with CATPCA. CATPCA 1115 is a technique derived from linear principal component analysis PCA that reduces a set of numerical variables to a lower number of noncorrelated components with the smallest loss.
The goal of principal components analysis is to reduce an original set of variables into a smaller set of uncorrelated components that. Linting M van der Kooij A. Child and Family Studies Leiden University The Netherlands.
Nonlinear principal components analysis with CATPCA. Nonlinear PCA available as the program CATPCA1 CATegorical Principal components analysis implemented in the Categories module of SPSS Meulman Heiser SPSS 1999 is an optimal scaling method belonging to the nonlinear multivariate analysis techniques. Nonlinear principal components analysis with CATPCA.
Up to 10 cash back To avoid this limitation categorical principal component analysis CATPCA or nonlinear principal component analysis NLPCA has been introduced as an alternative in dealing with nominal and ordinal data Linting et al. The authors describe it as a necessary step but I cant seem to find it within the CATPCA drop-down menu. Linting and Van der Kooij 2012.
Its aim is to reduce a larger set of variables into a smaller set of artificial variables called principal components which account for most of the variance in the original variables. Version 2 Principal component analysis PCA is a mainstay of modern data analysis - a black box that is widely used but poorly understood. Categorical principal components analysis is also known by the acronym CATPCA for categorical principal components analysis.
Nonlinear principal components analysis with CATPCA. The most important advantages of nonlinear over linear PCA are that it incorporates nominal and ordinal. One of the most commonly used methods to reduce the dimension of data and reveal hidden patterns is the principal component analysis PCA.
Nonlinear Principal Components Analysis PCA addresses the nonlinearity problem by relaxing the linear restrictions on standard PCA. Alternatively categorical also known as nonlinear principal components analysis CATPCA has been developed for the data given mixed measurement level such that nominal ordinal or numeric which may not have linear relationship with each other. This article is set up as a tutorial for nonlinear principal components analysis NLPCA systematically guiding the reader through the process of analyzing actual data on.
The nonlinear categorical principal component analysis. Journal of Personality. This method is the nonlinear equivalent of standard PCA and reduces the observed variables to a number of uncorrelated principal components.
This chapter focuses on the analysis of ordinal and nominal multivariate data using a special variety of principal components analysis that includes nonlinear optimal scaling transformation of the variables. Mariëlle Linting Anita van der Kooij. Relevant clinical information can influence the diagnostic probability and reporting of ultrasound findings.
Nonlinear Principal Components Analysis With CATPCA. Relationships among these potential explanatory variables the endpoint ICRH and the associated event time variables days until ICRH were examined by nonlinear categorical principal components analysis CATPCA evaluating the relative importance of the variables within their multivariable setting and the strength of their associations based. Begingroup You can save principal scores from the CATPCA procedure as new variables to your dataset and then analyse them as any other variables.
This method is the nonlinear equivalent of standard PCA and reduces the observed variables to a number of uncorrelated principal components. Principal components analysis PCA for short is a variable-reduction technique that shares many similarities to exploratory factor analysis. This article is set up as a tutorial for nonlinear principal components analysis NLPCA systematically guiding the reader through the.
The nonlinear categorical principal component analysis method CATPCA is an extended version of this method for categorical data ie ordinal and nominal data. The authors provide a didactic treatment of nonlinear categorical principal components analysis PCA. J Pers Assess 2012 94112-25.
Pdf Nonlinear Principal Components Analysis Introduction And Application Semantic Scholar
Pdf Nonlinear Principal Components Analysis Introduction And Application Semantic Scholar
Pdf Nonlinear Principal Components Analysis Introduction And Application Semantic Scholar
Pdf Principal Components Analysis With Nonlinear Optimal Scaling Transformations For Ordinal And Nominal Data Semantic Scholar
Pdf Principal Components Analysis With Nonlinear Optimal Scaling Transformations For Ordinal And Nominal Data Semantic Scholar
Pdf Nonlinear Principal Components Analysis Introduction And Application Semantic Scholar
Pdf Principal Components Analysis With Nonlinear Optimal Scaling Transformations For Ordinal And Nominal Data Semantic Scholar
0 comments
Post a Comment